Menu
- Objectives
- Past events under the sponsorship of IGLU
- Public Open Access Datasets, Software and Demonstrations
- Partners inside IGLU and list of institutional partners
- Contributors
- Publications
- Media
Objectives
Interactive Grounded Language Understanding is an ability that develops in young children through joint interaction with their caretakers and their physical environment. At this level, human language understanding could be referred as interpreting and expressing semantic concepts (e.g. objects, actions and relations) through what can be perceived (or inferred) from current context in the environment. Previous work in the field of artificial intelligence has failed to address the acquisition of such perceptually-grounded knowledge in virtual agents (avatars), mainly because of the lack of physical embodiment (ability to interact physically) and dialogue, communication skills (ability to interact verbally). We believe that robotic agents are more appropriate for this task, and that interaction is a so important aspect of human language learning and understanding that pragmatic knowledge (identifying or conveying intention) must be present to complement semantic knowledge. Through a developmental approach where knowledge grows in complexity while driven by multimodal experience and language interaction with a human, we propose an agent that will incorporate models of dialogues, human emotions and intentions as part of its decision-making process. This will lead anticipation and reaction not only based on its internal state (own goal and intention, perception of the environment), but also on the perceived state and intention of the human interactant. This will be possible through the development of advanced machine learning methods (combining developmental, deep and reinforcement learning) to handle large-scale multimodal inputs, besides leveraging state-of-the-art technological components involved in a language-based dialog system available within the consortium. Evaluations of learned skills and knowledge will be performed using an integrated architecture in a culinary use-case, and novel databases enabling research in grounded human language understanding will be released. IGLU will gather an interdisciplinary consortium composed of committed and experienced researchers in machine learning, neurosciences and cognitive sciences, developmental robotics, speech and language technologies, and multimodal/multimedia signal processing. We expect to have key impacts in the development of more interactive and adaptable systems sharing our environment in everyday life.
IGLU is part of the European CHIST-ERA network (http://www.chistera.eu/projects/iglu) .
The coordinator is prof. J. Rouat, NECOTIS, Université de Sherbrooke, Québec, Canada,
https://www.gel.usherbrooke.ca/rouat/.
Past events under the sponsorship of IGLU
First Master Class on Human Language Understanding in the framework of the CHIST-ERA HLU call , Paris, April 10-11th, 2018
Shared sponsorship with CHIST-ERA, M2CR, ReGROUND, ATLANTIS.
First Visually-Grounded Interaction and Language (ViGIL), NIPS 2017 Workshop
, Long Beach, California, USA, Friday, December 8th, 2017
Shared sponsorship with facebook research and google DeepMind.
First International Workshop on Grounding Language Understanding, Satellite of Interspeech 2017
, KTH Royal Institute of Technology, Stockholm, Sweden, Friday, August 25th, 2017
Shared sponsorship with ISCA.
Partners inside IGLU and list of institutional partners
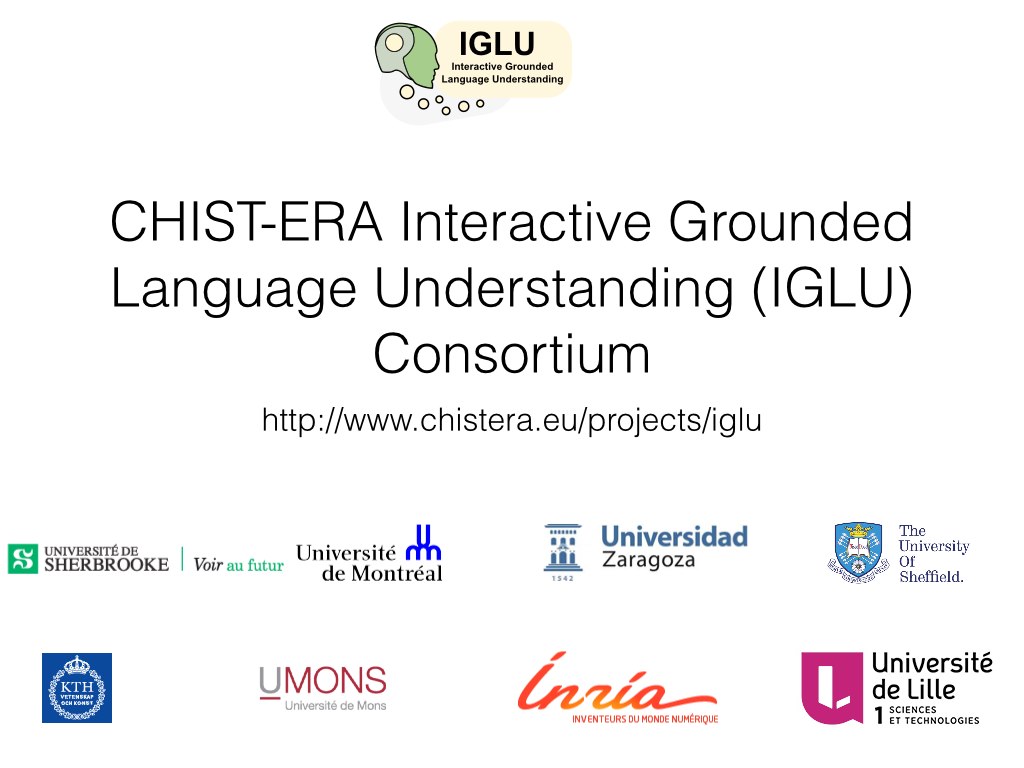
List of institutional partners
Partnership with DEVINE for a final integration on a humanoid robot.
Public Open Access Datasets, Software and Demonstrations
Databases
We built 6 databases that cover 6 levels of knowledge types and
representations giving a gradation in semantic representation and levels of
interactions and grounding:
-
A first one for environment representation and learning for a mobile platform ( ROS Create database, Documentation on arXiv );
-
A second one for object learning and representation on a Baxter platform (Multimodal Human-Robot Interaction (MHRI) database);
-
A third one for dialogue and a richer semantic with the new GuessWhat game (The GuessWhat?! database and software );
-
A fourth one for a larger variety in the audio inside the HoME environment ( Audio dataset for the HoME environment ). It is a collection of audio samples that we selected and processed from the Freesound.org collaborative database of Creative Commons Licensed sounds;
-
A fifth one for acoustic reasoning and question answering ( the CLEAR dataset ). It is composed of audio recordings and questions/answers about their content. The dataset was generated using the CLEAR software.
-
The last is an acoustical multichannel dataset composed of 11 event classes recorded at several realistic positions in two different rooms. The dataset comprises two types of sequences according to the number of events in the sequence. 2662 unilabel sequences and 2724 multilabel sequences are recorded corresponding to a total of 5.24 hours. (The SECL-UMONS database );
Software
-
Cooperative Visually Grounded Dialogue Game with a Humanoid robot ( Documentation from the DEVINE team )( Source code and Software from the DEVINE team ). The task, undertaken by a robot, is the question answering game GuessWhat?!. The robot interacts with a human player who performs the role of the oracle. The oracle selects an object in a visual scene without disclosing it to the robot. Playing the role of the guesser, the robot asks questions to the person to deduce which object was selected. The guesser’s questions are generated using the player’s previous answers and visual information. Once the robot has a strong belief, it guesses the object chosen by the player and physically points to it in the scene.
-
HoME, a Household Multimodal Environment, has been designed to enable artificial agents to learn as humans do, in an interactive, multimodal, and richly contextualized setting. It provides support for vision, audio, semantics, physics and interaction with objects and other agents inside a 3D environment.
-
Real-time GCC-NMF Blind Speech Separation and Enhancement is a software that eases the audio interaction through the separation and enhancement of sound sources.
-
Generation code for the CLEAR dataset (Compositional Language and Elementary Acoustic Reasoning)
Demonstrations and Applications
-
Cooperative Visually Grounded Dialogue Game with a Humanoid robot, a NIPS 2018 Demonstration (Partnership with DEVINE ). ( Paper Abstract )( Video ) Computer Code and Software .
-
Incremental Object Learning with few data on a Baxter robot: Demonstration on a Baxter Robot (by UNIZAR).
Contributors
The IGLU consortium is composed of 8 research teams, across 6 different countries. The project is a total effort of 325 person-months (PM).
Experts
- Deep learning & machine learning - A. Courville (MILA, UdeM), https://mila.umontreal.ca/
- Reinforcement learning - O. Pietquin, B. Piot (CRIStAL, Lille1 & DeepMind), http://www.univ-lille1.fr/
- Neurosciences and cognitive sciences - J. Rouat (NECOTIS, UdeS & UdeM), https://www.gel.usherbrooke.ca/necotis/, R.K. Moore (U. Sheffield)
- Robotics - P.Y. Oudeyer (INRIA), A.C. Murillo (UNIZAR), J. Civera (UNIZAR)
- Signal Processing (auditory, vision) and machine learning - J. Rouat (UdeS & UdeM), S. Dupont (U. Mons), G. Salvi (KTH)
- Human-Machine interaction - S. Dupont (U. Mons)
Students
11 PhD & 3 Msc.A
Publications
2023
- Abdelnour, J.; Rouat, J.; Salvi, G., NAAQA: A Neural Architecture for Acoustic Question Answering, IEEE Transactions on Pattern Analysis and Machine Intelligence, Volume: 45, Issue: 4, 01 April 2023 DOI: 10.1109/TPAMI.2022.3194311
2022
-
Brousmiche, M.; Rouat, J.; Dupont S., Multimodal Attention Fusion Network for Audio-visual Event Recognition, Information Fusion Journal, in Press.
-
Herranz-Celotti, L.; Rouat J., Surrogate Gradients Design, preprint, [arxiv] https://arxiv.org/abs/2202.00282
-
Celotti, L.; Brodeur, S.; Rouat, J., AriEL: Volume Coding for Sentence Generation, preprint submitted at ICLR 2022, OpenReview comments and evaluations (https://openreview.net/forum?id=qTTccuW4dja)
2021
-
Abdelnour, J.; Rouat, J.; Salvi, G, NAAQA: A Neural Architecture for Acoustic Question Answering, submitted to IEEE Tr. on PAMI, preprint [arxiv] https://arxiv.org/abs/2106.06147
-
Brousmiche, M.; Rouat, J.; Dupont S., Multi-level Attention Fusion Network for Audio-visual Event Recognition, preprint, [arxiv] https://arxiv.org/abs/2106.06736
2020
-
Brousmiche, M.; Rouat, J.; Dupont S., SECL-UMons Database for Sound Event Classification and Localization, ICASSP 2020 - 2020 IEEE International Conference on Acoustics, Speech and Signal Processing (ICASSP), Barcelona, Spain, 2020, pp. 756-760. DOI: 10.1109/ICASSP40776.2020.9053298
-
Celotti, L.; Brodeur, S.; Rouat, J., AriEL: Volume Coding for Sentence Generation, arxiv
-
M Brousmiche, S Dupont, J Rouat, Intra and Inter-modality Interactions for Audio-visual Event Detection, Proceedings of the 1st International Workshop on Human-centric Multimedia Analysis (HuMA’20), pp. 5–11, https://doi.org/10.1145/3422852.3423486
2019
-
A. Selamtz, A. Castellana, G. Salvi, A. Carullo, A. Astolfi, Effect of vowel context in cepstral and entropy analysis of pathological voices, Biomedical Signal Processing and Control, Volume 47, January 2019, Pages 350-357, DOI: 10.1016/j.bspc.2018.08.021
-
G. Saponaro, L. Jamone, A. Bernardino, G. Salvi, Beyond the Self: Using Grounded Affordances to Interpret and Describe Others’ Actions, IEEE Transactions on Cognitive and Developmental Systems, 2019, DOI: 10.1109/TCDS.2018.2882140
-
K. Stefanov, G. Salvi, Dimosthenis Kontogiorgos, Hedvig Kjellström, and J. Beskow, Modeling of Human Visual Attention in Multiparty Open-World Dialogues, ACM Transactions on Human-Robot Interaction, 2019, No. 8, DOI: 10.1145/3323231
-
K. Stefanov, J. Beskow, and G. Salvi, Self-Supervised Vision-Based Detection of the Active Speaker as Support for Socially-Aware Language Acquisition, IEEE Transactions on Cognitive and Developmental Systems, 2019. DOI: 10.1109/TCDS.2019.2927941
-
Brousmiche, M.; Rouat, J.; Dupont S., Audio-Visual Fusion And Conditioning With Neural Networks For Event Recognition, IEEE 29th International Workshop on Machine Learning for Signal Processing (MLSP), Pittsburgh, PA, USA, 2019, pp. 1-6, DOI: 10.1109/MLSP.2019.8918712
-
Abdelnour, J.; Salvi, G.; Rouat, J., From Visual to Acoustic Question Answering, arxiv
-
Wood, S. U.; Rouat, J.,Unsupervised Low Latency Speech Enhancement with RT-GCC-NMF, IEEE Journal of Selected Topics In Signal Processing 13 (2), 332-346, DOI: 10.1109/JSTSP.2019.2909193
-
Zhang, C.; Öztireli, C.; Mandt, S.; Salvi, G., Active Mini-Batch Sampling using Repulsive Point Processes, The AAAI Conference on Artificial Intelligence, 2019. arxiv
2018
-
Prince-Tremblay J.; Balafrej I.; Labelle F.; Martel-Denis F.; Matte E.; Chouinard-Beaupré J.; Létourneau A.; Mercier-Nicol A.; Brodeur S.; Ferland F.; Rouat J.; A Cooperative Visually Grounded Dialogue Game with a Humanoid Robot, NeurIPS 2018 demonstration, https://devineproject.github.io/abstract_devine.pdf
-
Golemo F., How to Train Your Robot - New Environments for Robotic Training and New Methods for Transferring Policies from the Simulator to the Real Robot, PhD thesis, 2018. [pdf]
-
Golemo F.; Taiga AA.; Courville A.; Oudeyer PY., Sim-to-Real Transfer with Neural-Augmented Robot Simulation, In Conference on Robot Learning, 2018. [pdf]
-
Celotti, L.; Brodeur, S.; Rouat, J.,Language coverage and generalization in RNN-based continuous sentence embeddings for interacting agents, ViGiL NeurIPS workshop, 2018 [pdf]
-
Abdelnour, J.; Salvi, G.; Rouat, J.,CLEAR: A Dataset for Compositional Language and Elementary Acoustic Reasoning, ViGiL NeurIPS workshop, 2018 [pdf]
-
Belgoum, Z.; Abdelnour, J.; Brodeur, S.; Rouat, J.,Audio dataset for Household Multimodal Environment (HoME), IEEE Dataport, 2018, [doi]
-
Azagra, P.; Murillo A.C.; Lopes M.; Civera J.,Incremental Object Model Learning from Multimodal Human-Robot Interactions, ViGiL NeurIPS workshop, 2018 [pdf]
-
Delbrouck, J.B.; Dupont, S., Object-oriented Targets for Visual Navigation using Rich Semantic Representations, ViGiL NeurIPS workshop, 2018 [pdf]
-
Abdelnour, J.; Salvi, G.; Rouat, J. CLEAR: A Dataset for Compositional Language and Elementary Acoustic Reasoning, arXiv:1811.10561v1, 2018
-
Delbrouck, J.B.; Dupont, S., Bringing back simplicity and lightliness into neural image captioning, arXiv:1810.06245v1, 2018.
-
Dumoulin, V.; Perez, E.; Schucher, N.; Strub, F.; Vries, H.; Courville, A.; Bengio, Y., Feature-wise transformations. In Proceedings Distill, 2018 pdf
-
Strub F.; Seurin, M.; Perez, E.; de Vries, H.; J. Mary; Preux, P.; Courville, A.; Pietquin, O., Visual Reasoning with Multi-hop Feature Modulation In Proceedings ECCV, 2018 pdf
-
Delbrouck, J.B.; Dupont, S., Umons submission for wmt18 multimodal translation task. In Proceedings of the Third Conference on Machine Translation, Brussels, Belgium. Association for Computational Linguistics, 2018 [pdf]
-
Brodeur, S.; Perez, E.; Anand, A.; Golemo, F.; Celotti, L.; Strub, F.; Rouat, J.; Larochelle, H. & Courville, A., HoME: a Household Multimodal Environment, ICLR 2018 Workshop Track, 6th International Conference on Learning Representations, https://openreview.net/forum?id=B1pJ3dkwG
-
Brodeur, S.; Carrier, S.; Rouat, J., CREATE: Multimodal Dataset for Unsupervised Learning and Generative Modeling of Sensory Data from a Mobile Robot, IEEE Dataport, 10.21227/H2M94J, 2018
-
Brodeur, S.; Carrier, S.; Rouat, J., CREATE: Multimodal Dataset for Unsupervised Learning, Generative Modeling and Prediction of Sensory Data from a Mobile Robot in Indoor Environments, CoRR, arXiv:1801.10214v1 [cs.RO], 2018
-
K. Stefanov, J. Beskow, G. Salvi, Self-Supervised Vision-Based Detection of the Active Speaker as a Prerequisite for Socially-Aware Language Acquisition, submitted to IEEE Transactions on Cognitive and Developmental Systems.
-
G. Saponaro, L. Jamone, A. Bernardino, G. Salvi, Beyond the Self: Using Grounded Affordances to Interpret and Describe Others’ Actions, in IEEE Transactions on Cognitive and Developmental Systems.
-
E. Perez, F. Strub, H. Vries, V. Dumoulin, A. Courville FiLM: Visual Reasoning with a General Conditioning Layer. arXiv preprint arXiv:1709.07871. Association for the Advancement of Artificial Intelligence 2018 (AAAI 2018), oral presentation.
2017
-
Delbrouck, J.B.; Dupont, S., Modulating and attending the source image during encoding improves Multimodal Translation, ViGiL NeurIPS workshop, 2018
-
Brodeur, S.; Perez, E.; Anand, A.; Golemo, F.; Celotti, L.; Strub, F.; Rouat, J.; Larochelle, H. & Courville, A. HoME: a Household Multimodal Environment, NIPS 2017’s Visually-Grounded Interaction and Language Workshop, 2017, https://github.com/nips2017vigil/nips2017vigil.github.io/raw/master/papers/2017/HoME_a_household.pdf, [arxiv] preprint https://arxiv.org/abs/1711.11017,
-
Pablo Azagra, Florian Golemo, Yoan Mollard, Ana Cristina Murillo and Javier Civera, A Multimodal Dataset for Object Model Learning from Natural Human-Robot Interaction, 2017 IEEE/RSJ International Conference on Intelligent Robots and Systems (IROS), Vancouver, BC, 2017, pp. 6134-6141. [pdf]
-
Pablo Azagra, Javier Civera, and Ana C. Murillo. Finding Regions of Interest from Multimodal Human-Robot Interactions, Proc. GLU 2017 International Workshop on Grounding Language Understanding. 2017. [pdf]
-
Julien Pérolat, Florian Strub, Bilal Piot, Olivier Pietquin, Learning Nash Equilibrium for General-Sum Markov Games from Batch Data. arXiv preprint arXiv:1606.08718, Accepted at the International Conference on Artificial Intelligence and Statistics 2017 (AIStat 2017)
-
Brodeur, S. & Rouat, J., Optimality of Inference in Hierarchical Coding for Distributed Object-Based Representations, 15th. IEEE Canadian Workshop on Information Theory (CIWT), DOI:10.1109/CWIT.2017.7994828, [pdf]
-
Harm de Vries, Florian Strub, Sarath Chandar, Olivier Pietquin, Hugo Larochelle, Aaron Courville, GuessWhat?! Visual object discovery through multi-modal dialogue. arXiv preprint arXiv:1611.08481, Accepted at the Conference on Computer Vision and Pattern recognition 2017 (CVPR 2017) - Spotlight
-
Wood, S.U.; Rouat, J.; Dupont, S. & Pironkov, Blind Speech Separation and Enhancement with GCC-NMF. IEEE Transactions on Audio, Speech and Language Processing, pp. 3329-3341, 2017, DOI:10.1109/TASLP.2017.2656805, [pdf]
-
S. U. N. Wood and J. Rouat, Real-time speech enhancement with GCC- NMF. Proc. Interspeech 2017, 2665-2669, DOI: 10.21437/Interspeech.2017-1458.
-
S. U. N. Wood and J. Rouat, Real-time speech enhancement with GCC-NMF: Demonstration on the Raspberry Pi and NVIDIA Jetson. Proc. Interspeech 2017, 2048-2049, [pdf]
-
S. U. N. Wood and J. Rouat, Towards GCC-NMF speech enhancement for hearing assistive devices: Reducing latency with asymmetric windows 1st Int. Conference on Challenges in Hearing Assistive Technology (CHAT-17), Stockholm, Sweden, August 19, 2017, [pdf]
-
F. Strub, H. de Vries, J. Mary, B. Piot, A. Courville, O. Pietquin, End-to-end optimization of goal-driven and visually grounded dialogue systems.. arXiv preprint arXiv:1703.05423, Accepted at the International Joint Conference in Artificial Intelligence 2017 (IJCAI 2017) - Oral presentation.
-
Kumar Dhaka, A., Salvi, G., Sparse Autoencoder Based Semi-Supervised Learning for Phone Classification with Limited Annotations, Proc. GLU 2017 International Workshop on Grounding Language Understanding, Stockholm, Sweden, 22-26, DOI: 10.21437/GLU.2017-5.
-
Fahlström Myrman, A., Salvi. G., Partitioning of Posteriorgrams Using Siamese Models for Unsupervised Acoustic Modelling, Proc. GLU 2017 International Workshop on Grounding Language Understanding, Stockholm, Sweden, 27-31, DOI: 10.21437/GLU.2017-6.
-
Delbrouck, J., Dupont, S., Seddati, O., Visually Grounded Word Embeddings and Richer Visual Features for Improving Multimodal Neural Machine Translation. Proc. GLU 2017 International Workshop on Grounding Language Understanding, 62-67, DOI: 10.21437/GLU.2017-13
-
Stefanov, K., Beskow, J., Salvi, G., Vision-based Active Speaker Detection in Multiparty Interaction, Proc. GLU 2017 International Workshop on Grounding Language Understanding, Stockholm, Sweden, 47-51, DOI: 10.21437/GLU.2017-10.
-
Saponaro, G., Jamone, L., Bernardino, A., Salvi, G., Interactive Robot Learning of Gestures, Language and Affordances, Proc. GLU 2017 International Workshop on Grounding Language Understanding, Stockholm, Sweden, 83-87, DOI: 10.21437/GLU.2017-17.
-
Brodeur, S., Celotti, L., Rouat, J., Proposal of a Generative Model of Event-based Representations for Grounded Language Understanding, Proc. GLU 2017 International Workshop on Grounding Language Understanding, Stockholm, Sweden, 68-72, DOI: 10.21437/GLU.2017-14.
-
Delbrouck, J., Dupont, S., An empirical study on the effectiveness of images in Multimodal Neural Machine Translation, Proceedings of the 2017 Conference on Empirical Methods in Natural Language Processing
-
H. de Vries , F. Strub , J. Mary, H. Larochelle, O. Pietquin, A. Courville, Modulating Early visual Processing by language. arXiv preprint arXiv:1707.00683, Accepted at the Conference on Neural Information Processing System 2017 (NIPS 2017) - Spotlight
-
Delbrouck, J., Dupont, S., Multimodal Compact Bilinear Pooling for Multimodal Neural Machine Translation, arXiv preprint arXiv:1707.03017
-
E. Perez, H. Vries, F. Strub, V. Dumoulin, A. Courville, Learning Visual Reasoning Without Strong Priors. arXiv preprint arXiv:1707.03017, Accepted at ICML Speech and Language Processing Workshop 2017.
2016
-
Pablo, A., Mollard, Y., Golemo, F., Murillo, A. C., Lopes, M., & Civera, J. (2016, December). A Multimodal Human-Robot Interaction Dataset. In Future of Interactive Learning Machines Workshop, NIPS 2016. Barcelona, Spain. December 2016, http://www.filmnips.com/accepted-papers/, [pdf]
-
Cambra, A. B., Muñoz, A., Guerrero, J. J., & Murillo, A. C. Dense Labeling with User Interaction: An Example for Depth-Of-Field Simulation. British Machine Vision Conference (BMVC), 2016.
Media
-
5 March 2018, article in “Les nouvelles” , a French publication from Université de Sherbrooke
-
12 October 2017, article in “La Presse” , a French journal from Montréal
-
October 2017, French INRIA jubilee , France (in preparation)